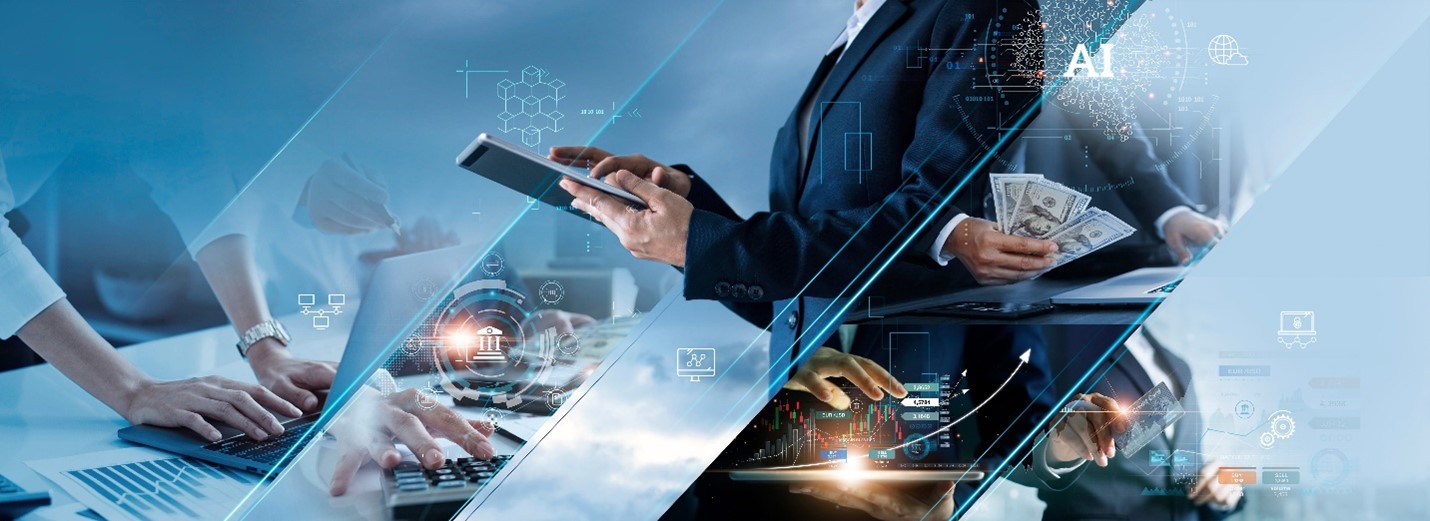
Pablo Salame Explores The Role of Artificial Intelligence in Modern Portfolio Management: Transforming Risk Analysis and Investment Optimization
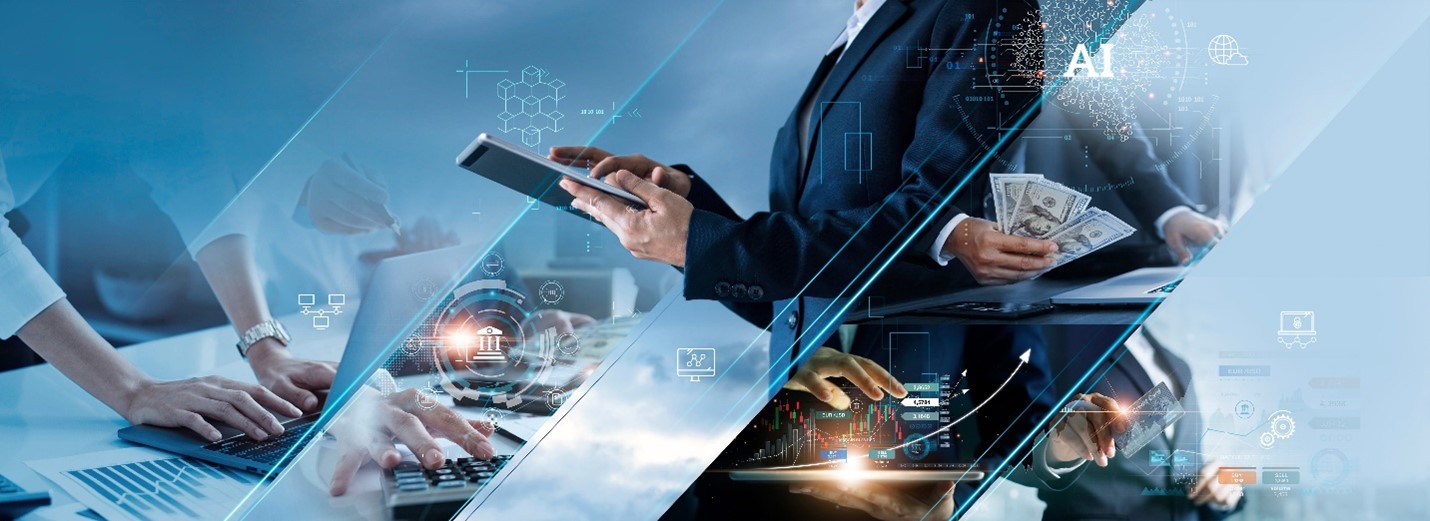
In recent years, artificial intelligence (AI) has emerged as a game-changer across various industries, revolutionizing the way professionals approach complex challenges. Nowhere is this transformation more apparent than in the field of portfolio management. Traditionally reliant on statistical analysis, human expertise, and market intuition, portfolio management is undergoing a radical shift due to the integration of AI and machine learning (ML) technologies. From risk analysis to investment optimization, AI-driven tools are redefining the ways in which asset managers, financial advisors, and individual investors make investment decisions, leading to enhanced efficiency, increased accuracy, and better returns.
In this article, Pablo Salame explores the profound impact of AI on modern portfolio management, highlighting its role in risk analysis, asset allocation, and the customization of investment strategies.
Understanding AI and Machine Learning in Finance
Before diving into the specifics, it’s essential to understand the distinctions between AI and ML and their applications in finance. Pablo Salame explains that AI refers to the development of systems capable of performing tasks that would normally require human intelligence, such as data analysis, decision-making, and pattern recognition. Machine learning, a subset of AI, involves training algorithms to learn from data and improve over time without explicit programming. When applied to finance, AI and ML are capable of processing vast amounts of financial data, identifying patterns, predicting outcomes, and automating complex tasks—all in real-time.
In portfolio management, these capabilities translate into more accurate predictions of market trends, personalized investment recommendations, and dynamic asset allocation strategies. AI helps investors understand and manage risk more effectively, optimize returns, and streamline portfolio oversight.
AI-Driven Risk Analysis
Risk management is at the heart of portfolio management, as investors seek to maximize returns while minimizing potential losses. Pablo Salame of NYC understands that traditionally, risk analysis relied on historical data, financial ratios, and human expertise. However, these conventional methods have limitations, particularly in accounting for rapidly changing market conditions or complex risk factors. AI and ML are enhancing risk analysis by leveraging vast data sources and identifying correlations that may be overlooked by human analysts.
- Enhanced Data Processing: AI systems can process massive amounts of structured and unstructured data in real time, including financial statements, news articles, economic indicators, and even social media sentiment. Pablo Salame explains that this capability enables a more comprehensive view of potential risk factors, providing deeper insights into market volatility, geopolitical events, and sector-specific risks.
- Predictive Analytics: Machine learning algorithms excel at predicting market movements based on historical trends and real-time data inputs. These predictive models help portfolio managers anticipate risk with a high degree of accuracy. For instance, AI-powered algorithms can detect early warning signals of potential downturns, allowing managers to make timely adjustments to their portfolios.
- Scenario Analysis and Stress Testing: AI can simulate various market conditions and stress-test portfolios to assess how they would perform in extreme scenarios. This ability to quickly analyze different market environments is invaluable for risk management, enabling portfolio managers to prepare for and mitigate potential losses under adverse conditions.
Investment Optimization and Asset Allocation
In addition to risk analysis, AI is revolutionizing how portfolio managers approach investment optimization and asset allocation. Pablo Salame understands that traditional optimization models rely on historical data and assumptions about market behavior, but these approaches can be rigid and may not account for the nuanced dynamics of modern financial markets. AI-driven optimization models, by contrast, can adjust dynamically, learning from new data to refine strategies continually.
- Dynamic Asset Allocation: Machine learning algorithms can evaluate thousands of variables and correlations to determine the ideal mix of assets for a given portfolio. Unlike traditional methods, AI-driven asset allocation models can adapt to market shifts in real-time, allowing portfolios to be rebalanced in response to evolving economic conditions. Pablo Salame of NYC explains that this dynamic approach enables portfolio managers to capitalize on emerging opportunities and avoid overexposure to high-risk assets.
- Diversification with Granular Precision: AI-driven asset allocation models allow for a more granular analysis of diversification. Pablo Salame emphasizes that by examining correlations between assets on a deeper level, AI can identify assets with low or negative correlations, providing more effective diversification and reducing overall portfolio risk. This approach is particularly beneficial in managing portfolios with high degrees of complexity, such as those that include alternative assets or exposure to multiple international markets.
- Customization and Personalization: AI-powered systems can create customized portfolios tailored to an investor’s specific risk tolerance, time horizon, and financial goals. This level of personalization was previously limited to high-net-worth individuals with access to private wealth managers. Now, with AI, even retail investors can access personalized portfolio recommendations that align closely with their unique financial objectives.
Automation in Portfolio Management
The automation of portfolio management processes is another area where AI is making a significant impact. Routine tasks, such as rebalancing portfolios, monitoring performance, and executing trades, can be automated using AI-driven systems. Pablo Salame explains that this reduces the workload on human managers and ensures a consistent, disciplined approach to portfolio management.
- Trade Execution and Rebalancing: AI algorithms can execute trades automatically based on predefined criteria or in response to market signals, ensuring that portfolios are rebalanced without delay. Pablo Salame of NYC explains that this is especially valuable in volatile markets, where rapid response times can make a substantial difference in performance. Automated rebalancing also ensures that portfolios remain aligned with an investor’s risk tolerance and investment goals.
- Continuous Monitoring and Reporting: AI-driven platforms can monitor portfolio performance and generate reports in real-time, providing portfolio managers and clients with up-to-date information on returns, risk metrics, and other key indicators. Pablo Salame explains that this transparency fosters trust and enables data-driven decision-making.
- Cost Efficiency and Accessibility: By automating routine tasks, AI reduces the time and resources required for portfolio management. This increased efficiency lowers costs for investors and makes professional portfolio management more accessible to a broader audience. Robo-advisors, for example, leverage AI to offer low-cost, automated portfolio management services to retail investors who may not have the means to work with traditional financial advisors.
Challenges and Considerations
While AI offers numerous advantages in portfolio management, it is not without challenges. Data quality and transparency are critical to the accuracy of AI models; if the data used to train algorithms is biased or incomplete, the resulting predictions may be flawed. Additionally, AI models are complex, and even experienced portfolio managers may struggle to interpret the “black box” nature of some machine learning algorithms. Regulatory compliance is another consideration, as financial regulators are closely monitoring the use of AI in finance to ensure that automated systems are used responsibly and ethically.
The Future of AI in Portfolio Management
As AI technology continues to evolve, its role in portfolio management will only become more prominent. Advanced AI systems are likely to become even better at interpreting unstructured data, such as news articles and social media sentiment, which will enable more accurate predictions of market trends. Additionally, the growing integration of AI with other emerging technologies, such as blockchain and quantum computing, has the potential to further enhance portfolio management capabilities.
For investors and portfolio managers willing to embrace AI, the opportunities are vast. Pablo Salame of NYC emphasizes that by leveraging AI-driven insights, investors can build more resilient, adaptive portfolios that respond swiftly to market changes, ultimately leading to better investment outcomes. As the field of AI advances, it is likely that portfolio management will continue to evolve, driven by data-driven insights, automation, and increasingly sophisticated algorithms. In this new era, the combination of human expertise and AI-powered technology promises to unlock unprecedented levels of value for investors worldwide.